A 7-Step Guide to Setting Up a Successful Digital Marketing Experiment
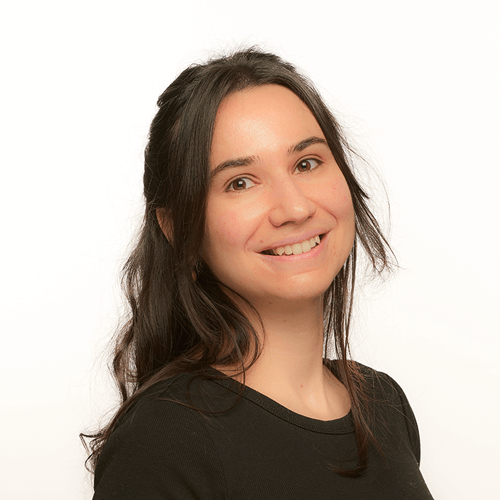
Ozlem Senlik Staff Data Scientist Airship
Digital marketing is a dynamic and ever-evolving field, but the key to staying ahead lies in continuous improvement. One of the most effective methods for improving digital marketing strategies is through well-designed experiments. These can uncover valuable insights, which can help to optimize your campaigns and drive better results. However, the success of an experiment hinges on careful planning and execution.
From the outset, it’s crucial to be clear about what you aim to learn from your experiment. Next, consider how you can quantify your learnings and success through measurable metrics. These metrics will serve as benchmarks to evaluate the effectiveness of your experiment.
It’s also important to understand your company’s tolerance for errors. Experiments inherently carry risks, and being aware of how much uncertainty your business — or decision-making process — can handle is vital for setting realistic expectations. Additionally, consider the ethical and legal implications of your experiment. Ensure that your experiment complies with data privacy laws and maintains high ethical standards to protect participants’ rights and privacy.
Here’s a comprehensive step-by-step guide to setting up a successful digital marketing experiment:
1. Define a Clear Hypothesis
The first step in any good experiment is to develop a clear and specific hypothesis. This hypothesis should be measurable, testable and focused on a particular aspect of your digital marketing campaign. For example, instead of saying, “We want to improve our email click-through rates,” you should say, “We hypothesize that changing the subject line of our email will improve our click-through rates by 10%.”
2. Identify Valid Metrics
The metrics used to evaluate the results of your experiment should be valid, relevant and aligned with your hypothesis. For example, if you’re testing a new landing page, you might measure the conversion rate or the bounce rate. Some other standard metrics include return on investment (ROI) and customer acquisition cost (CAC). It’s important to ensure that the data collected is both quantitative and qualitative and that you can compare the results against the control group.
3. Create a Control Group
It’s essential to compare the results of your experiment against a baseline, which is what a control group provides. The control group should be similar to the test group but not receive the treatment being tested. Instead, it should receive a standard treatment with a known effect or no treatment at all, which serves as the initial baseline. For instance, if you introduce your first ad, the control group should not be exposed to any ads — enabling you to determine the ad’s effectiveness and justify further ad expenditures.
You can further enhance outcomes by testing a new ad against the first one. In this scenario, the control group should be exposed to the first ad, while the test group sees the new ad. Consider utilizing Airship’s global holdout group to measure your initial baseline and establish marketing’s true lift across the metrics you’re interested in if you haven’t done so already. You can also use Campaign Category holdout groups to exclude specific campaigns to determine their impact on onboarding, abandoned carts, product rating requests and conversion rates.
4. Randomize Your Sample
Randomization is another critical factor in a good experiment. The test and control groups should be selected randomly to ensure that any differences observed are due to the treatment and not other factors — helping to eliminate bias and increase the reliability of your results. For example, if you’re testing a new email campaign, randomly select a portion of your email list to receive the new campaign while the remaining portion receives the old campaign. Airship’s experimentation platform helps you automatically generate randomized experiment groups.
5. Determine Sample Size
The required sample size for your experiment is closely linked to your business’s error tolerance and the agility of your decision-making process. These are quantified as statistical factors, such as the significance level and the minimum effect size, which are then used to determine the necessary sample size through statistical power analysis. To find the optimal balance between error tolerance and agility, it’s essential to understand these key statistical factors. Once you do, you can use freely available sample size calculators to determine the required sample size for your experiment.
6. Apply Rigorous Analysis
The results of your experiment should be analyzed using appropriate statistical methods to determine the significance of your findings. It’s important to ensure that the statistical tests are appropriate for your data and hypothesis. Some standard statistical tests are Z-Test, T-Test and Chi-Square Test. You should also consider and control for any potential confounding variables in your analysis to ensure reliable and valid results.
7. Ensure Ethical and Legal Compliance
When designing an experiment, it’s crucial to take into account both legal and ethical considerations to ensure that the experiment is conducted responsibly and in compliance with regulations. Here are some key considerations:
- Data Privacy Laws:
- GDPR (General Data Protection Regulation): Businesses operating in or targeting users in the European Union must comply with GDPR, which governs how personal data is collected, processed, and stored.
- CCPA (California Consumer Privacy Act), MHMD (Washington’s My Health My Data Act), and other US state privacy laws: Businesses targeting users in various US states must comply with evolving state privacy laws applicable based on the user’s state of residence, such as the CCPA or MHMD. These laws include requirements to be transparent about data collection practices and to offer users options to opt out and delete their data.
- Other Regional Laws: Ensure compliance with other relevant data protection regulations, such as Canada’s PIPEDA or Brazil’s LGPD.
- Informed Consent: Before collecting data, obtain clear and explicit consent from customers. Your privacy policy and consent mechanisms should encompass what experiment data will be collected and how it will be used, but if a specific test requires something more you will need to explain the explicit purpose to participants and gain additional permissions. Similarly, use only the minimum data needed for the purpose of the experiment.
- Terms of Service: Ensure that your experiment adheres to the terms of service of any platforms or tools you are using (e.g., social media platforms, email service providers).
- Disclosure and Transparency: If the experiment involves influencers, paid promotions or endorsements, clearly disclose these relationships to comply with advertising standards and guidelines.
- Respect for Participants: Treat participants with respect and avoid any practices that could be considered manipulative or deceptive.
- Avoiding Harm: Design experiments in a way that minimizes potential harm to participants. This includes avoiding sensitive topics that could cause distress or discomfort.
- Data Security: Implement robust data security measures to protect participants’ personal information from unauthorized access or breaches.
- Anonymity and Confidentiality: Ensure that participants’ data is anonymized or kept confidential where appropriate, especially if sensitive information is involved.
- Bias and Fairness: Avoid introducing biases into the experiment that could unfairly disadvantage certain groups. Ensure that the sample population is representative and that the results are interpreted fairly.
- Participant Autonomy: Allow participants to opt out of the experiment and have their data deleted at any time, ensuring they have control over their data. Provide clear instructions on how they can withdraw their consent or request their data be deleted, if they choose to do so.
- Honesty and Integrity: Report the findings of the experiment honestly, even if the results do not support the desired outcome. Avoid manipulating data or misrepresenting results.
Experimentation is a powerful tool for improving your digital marketing campaigns, but it’s important to conduct experiments that are well-designed and scientifically rigorous. Following the key steps outlined above will go a long way to ensuring that your experiments are valid, reliable and useful for improving your digital marketing strategies.
Read related experimentation posts:
Subscribe for updates
If the form doesn't render correctly, kindly disable the ad blocker on your browser and refresh the page.