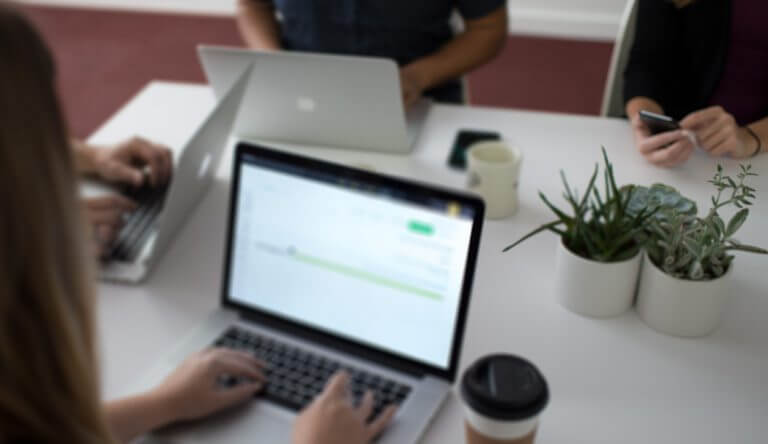
How to Use Machine Learning Data to Reduce Churn & Boost Engagement – Webinar Recap
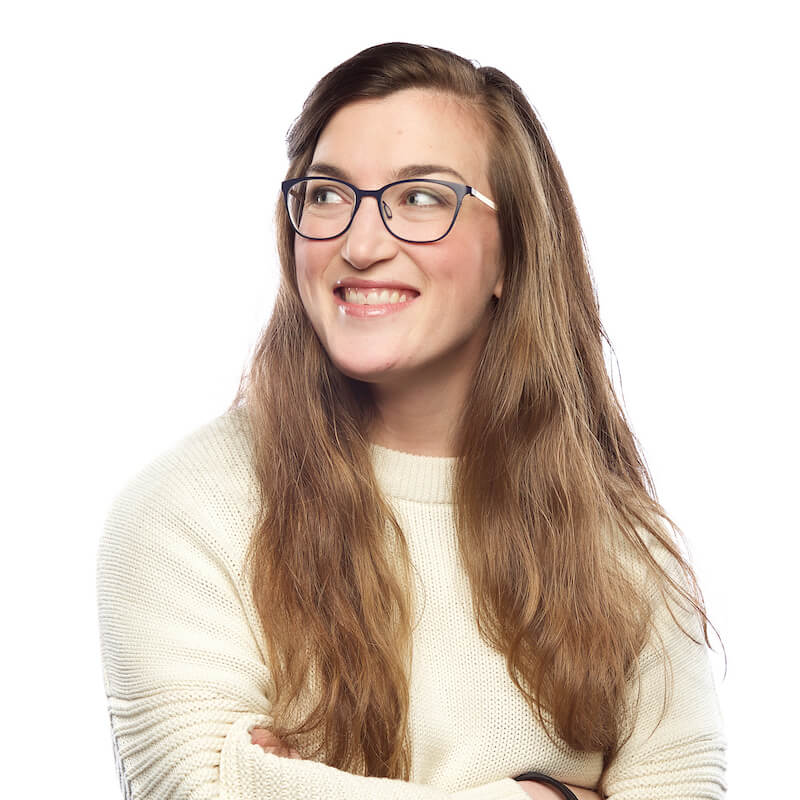
Lisa Orr Senior Product Manager
Data is a crucial tool for marketers, with 51% of marketers saying that using data to drive decision making is a high priority. AI can be a great tool to harvest, understand and use data but only 25% of marketers said they were pursuing AI acquisition and in a separate survey, 14% said they weren’t totally sure what AI was or could do.
To help marketers get a clearer idea on what AI can do for them today, Airship’s Lead Data Scientist, Lisa Orr, and Strategic Account Manager, Phil West, hosted a webinar on how to use data and our machine learning solutions, Predictive Churn and Predictive Send Time Optimization. We shared best practices and strategies from top brands who are using predictive data to reduce customer churn, improve engagement by 10% or more and drive increased customer lifetime value.
Here are the highlights from the webinar “How Top Brands Use Machine Learning Data to Reduce Churn & Boost Engagement (And How You Can Too)” — to get all of our insights, watch the full replay anytime.
1) The Differences Between AI, Machine Learning and Predictive Analytics
AI, Machine Learning and Predictive Learning are often put together and sometimes mistaken for each other, but they are three different tools that can help marketers make decisions based on data. To help differentiate between the three, we used the example of how a Roomba works.
AI is a branch of computer science dealing with the simulation of intelligent behavior in computers; the capability of a machine to imitate intelligent human behavior. A Roomba can appear intelligent, as if it’s making its own decisions wandering around, cleaning the dirt on your floor, but it’s actually following a set of rules (like turning after hitting a wall).
Machine Learning is a branch of artificial intelligence in which a computer generates rules based on raw data that has been fed into it, or put simply: a machine that learns from data. For a Roomba, beyond a simple set of rules (like turning after hitting a wall), the vacuum robot could be mapping the space, figuring out where it’s been so it doesn’t revisit the same places again.
Predictive Analytics is a variety of statistical techniques that analyze current and historical facts to make predictions about the future or otherwise unknown events and allow assessment of risk or potential associated with a particular set of conditions. This is a catchall phrase that ultimately means there is some smart data processing going on under the hood (like with the Roomba) providing marketers with a tool for making data-driven decisions fast.
2) Segment Audiences Based on Churn Risk to Boost Results
Airship built a powerful machine learning model that uses app data to predict churn, and simplifies the concept into three groups: High, Medium, and Low. Here’s our advice on how to approach each risk group:
High Risk: You’ve spent the money to acquire and engage, but now they’re at risk: how do you bring them back? Don’t be afraid to engage with them on a different channel. Everyone likes a deal, so sending a deal or offer to high-risk users to bring them back works well with email, but it also delivers results with the app. One Airship customer set up an automation pipeline for their high-risk group and that worked so well, it became a top-three revenue driver.
Medium Risk: Keep these users educated and engaged. Using educational or inspirational messages can remind them why they downloaded the app in the first place. You can also highlight features that low-churn users are using, to drive that same behavior with them. This is a strategy that also works with High-Risk users.
Low Risk: These are your really active folks and your best users, so how do you reward them or drive additional value? Since they are super active with the app, they are a great group to test different messages. You can try an additional push and see if they opt-out or delete the app with that extra message, but more often than not, the additional push actually helps the users engage with the app, not push them away.
3) Segment Your Audience to Send Test Notifications
No one wants to spam their customer, but you also want to make sure that you’re sending the right amount of notifications to get the best engagement. Segmenting your audience by risk can also separate the right audience to test those additional pushes.
A good test is to target low-risk, and possibly medium-risk, with an additional push, and see how the messages engage with that audience. What we see is that the additional push increases engagement, rather than push customers away into opting-out. One of our clients targeted a low-risk audience for an additional message every week and it became their best performing engagement.
It’s also important to think about consistency, rather than simply frequency. Another customer noticed a dramatic fall in their app opens and they weren’t sure why, until they realized they forgot to send their one weekly push. It can be easy to take notifications for granted!
4) The Best Time to Send a Notification May Surprise You
When is the best time to send a notification? It depends not only on the brand, but also by user. Sending a message during a time an individual is most likely to engage has a positive impact on direct open rates, and that window of time may be outside daytime hours. For one brand, Airship’s Send Time Optimization data showed that the highest engagement hours were actually the late night hours, with high activity happening even between 1 a.m. and 4 a.m.
Another example is a high-end retailer whose data showed a huge spike at night with opens, between 10 p.m. and midnight. Thinking about what kind of customers were using the app at that time (perhaps opening the app at the end of a long work day) they decided to try out inspirational messages, with the option of sending it out to everyone or targetting just those using the app that that time.
What works for some, may not work for all. Lisa worked with a data analyst that had a global audience to take into consideration. Before Send Time Optimization, he had to do a lot of research and make a lot of guesses, thinking about the different geographical regions and activity patterns of his customers. The Optimization button took away all that decision making, instead using data to send messages at the best times for the specific groups of customers. It worked so well, he sent a thank you note to the Airship team!
Is your brand using machine learning to reduce churn and boost engagement? For more insight and use cases, watch the webinar here and make sure to subscribe to our blog newsletter to get the best of Airship straight to your inbox.
Are you making smart data moves? Airship can help you develop strategies around Predictive Data that maximize your audience potential and engagement. Connect with us today and let’s talk!
Subscribe for updates
If the form doesn't render correctly, kindly disable the ad blocker on your browser and refresh the page.
Related Posts
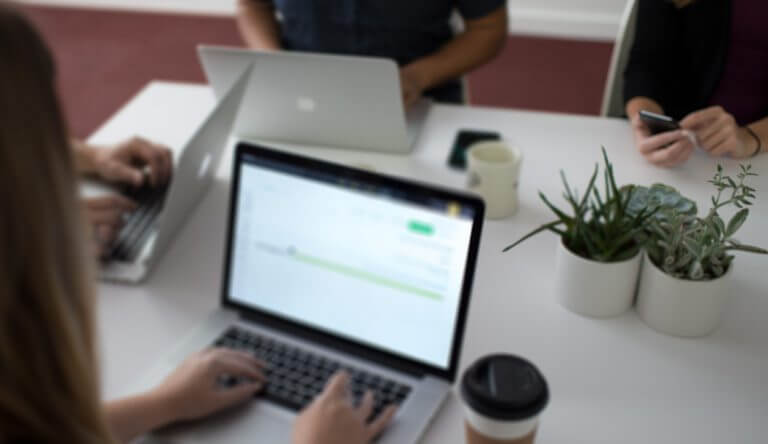
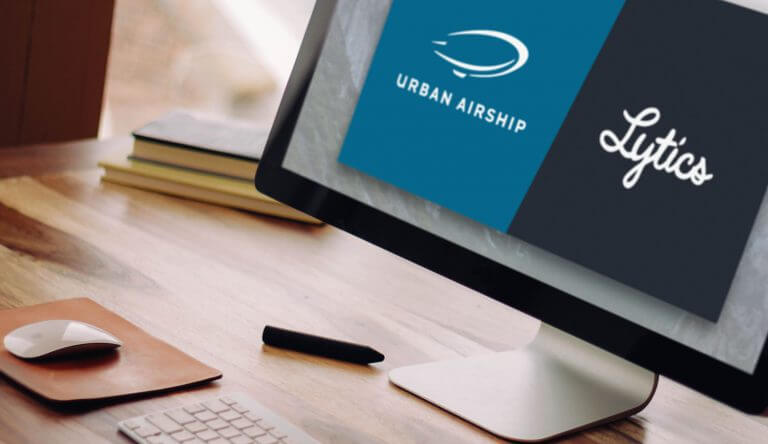
The Interplay of Data and Personalization
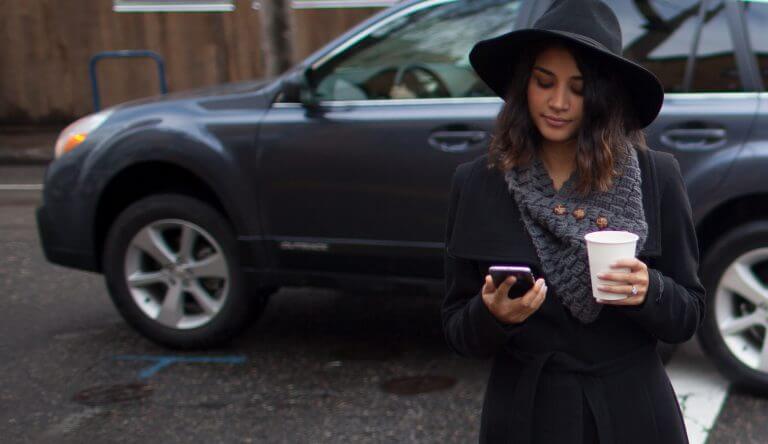